.jpg)
Indistinguishable from Magic: A River of Inquiry into AI
“Any sufficiently advanced technology is indistinguishable from magic” - Arthur C. Clarke
Over the past 12 months, the public release of several LLMs (Large Language Models) and chat interfaces has left us all feeling like we are experiencing, and in many ways conjuring, magic.
But how do we effectively invest in a technology that moves quickly and in markets that are experiencing swift disruption? How do we disaggregate hype from reality? How do we support and advise our portfolio companies?
In this 4-part series we explore:
Part 1: State of AI - a high-level recap of what has happened during the breakout year of AI.
Part 2: Landscape - a review of the AI landscape and where the emerging pockets of opportunity for investment are.
Part 3: Investment Thesis - an overview of our mental model for assessing AI companies.
Part 4: Company Spotlights - case studies from within our portfolio and noteworthy external examples.
Part 1: State of AI
Over recent decades, we have seen three major phases of AI.
Pre and during the 2000s, AI was underscored by rule-based systems. Systems built on top of a set of manually created classification rules. For example, a set of rules related to classifying animals:
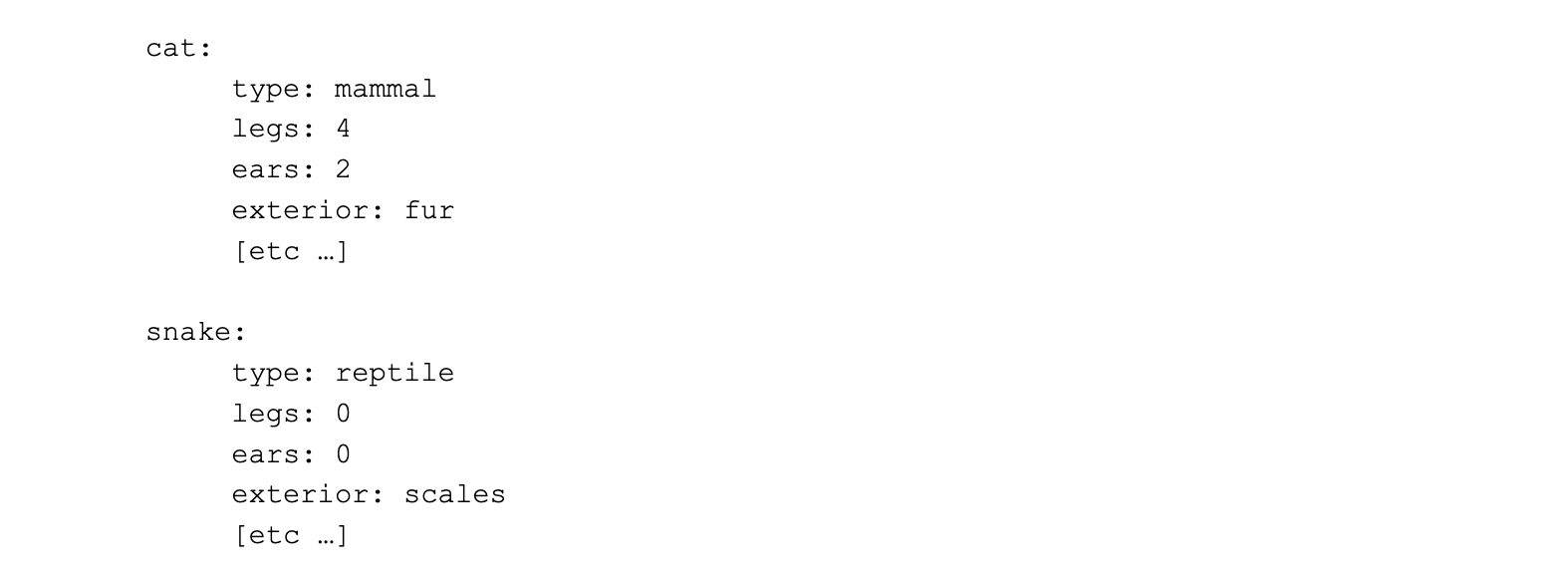
Insert an image, and these systems would process it through a set of rules and respond, “this is a cat”.
The world is a lot more complex than a simple set of rules. Dogs are also mammals with 4 legs, 2 ears, and fur, and so these systems are easily fallible.
In the 2010s, predictive neural networks became more viable through scale advancements in compute power.
Neural networks could handle ‘messier’ pattern recognition tasks. We could train a model on a volume of cat images and it would learn their pixel patterns to more easily identify a cat in other images.
This shift from ‘rules’ to ‘patterns’ was a step change but it remained constrained to narrow concepts and specific tasks.
The 2020s have been defined by generative AI and LLMs. For the first time, AI has gone mainstream.
Generative AI saw a shift from ‘supervised learning’, where datasets were defined, data was labelled, and models were pointed at specific tasks, to ‘self-supervised learning’ where models were trained on enormous unstructured datasets, and could process a seemingly endless number of tasks.
LaMDA for example, was trained on 1.5 trillion words, equivalent to a 74-story high stack of paper! When you poke and prod these models, they seem capable of answering any question with foundational intelligence.
The breakout year
The pace of innovation in AI this past year is unlike anything we’ve ever seen.
With mobile or cloud services, advancements were measured in years.
With Web 3, advancements were measured in months.
But with AI, we operate in weeks.
We’ve seen the efficiency of LLMs increase 100x at the implementation level. GPT4 now achieves 90th percentile marks in the Uniform Bar Exam, solves 78% of Math Olympiad questions, and reads text at thousands of words per second with no mental decay over time.
Open source communities have rallied like never before and most major enterprises have mobilised, with 21% of CEOs in Q1 2023 mentioning AI in their earnings calls.
Utility isn’t a promise, it’s real. GitHub Copilot is allowing many developers to code 60% faster, Midjourney helped artist Jason Allen take first place at the Colorado State Fair, and ChatGPT gained 100M MAUs in 60 days becoming the fastest-growing application of all time.
By comparison, it took Tik Tok 9 months and Instagram 2.5 years to reach the same milestone.
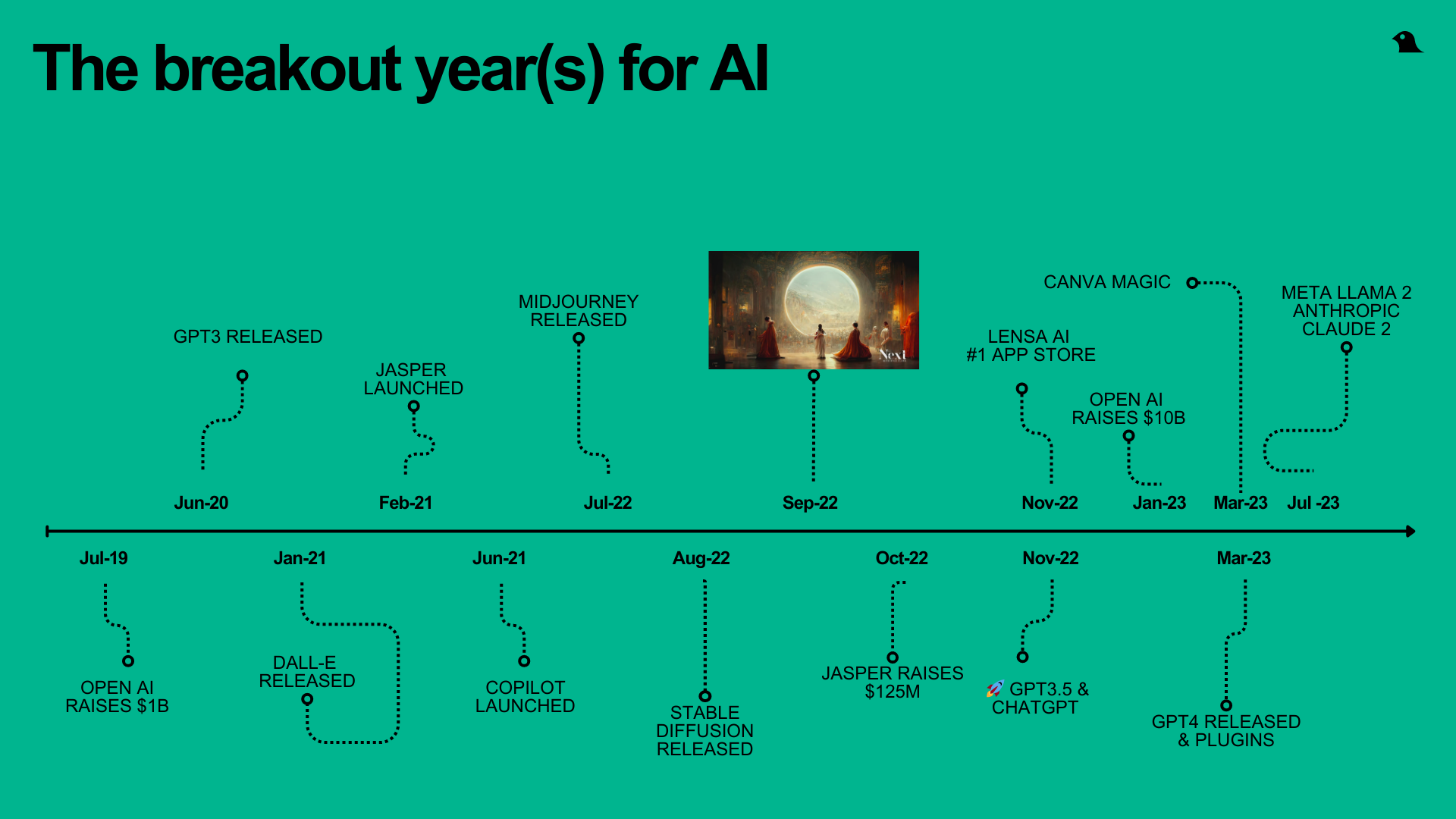
Investment interest has paired closely with this technical ramp. In Q1 2023, 50 startups raised US$12.5 billion in capital.
And in an otherwise passive M&A market, June 2023 saw MosaicML exit to Databricks for $1.3 billion and CaseText to Thomson Reuters for $650 million.
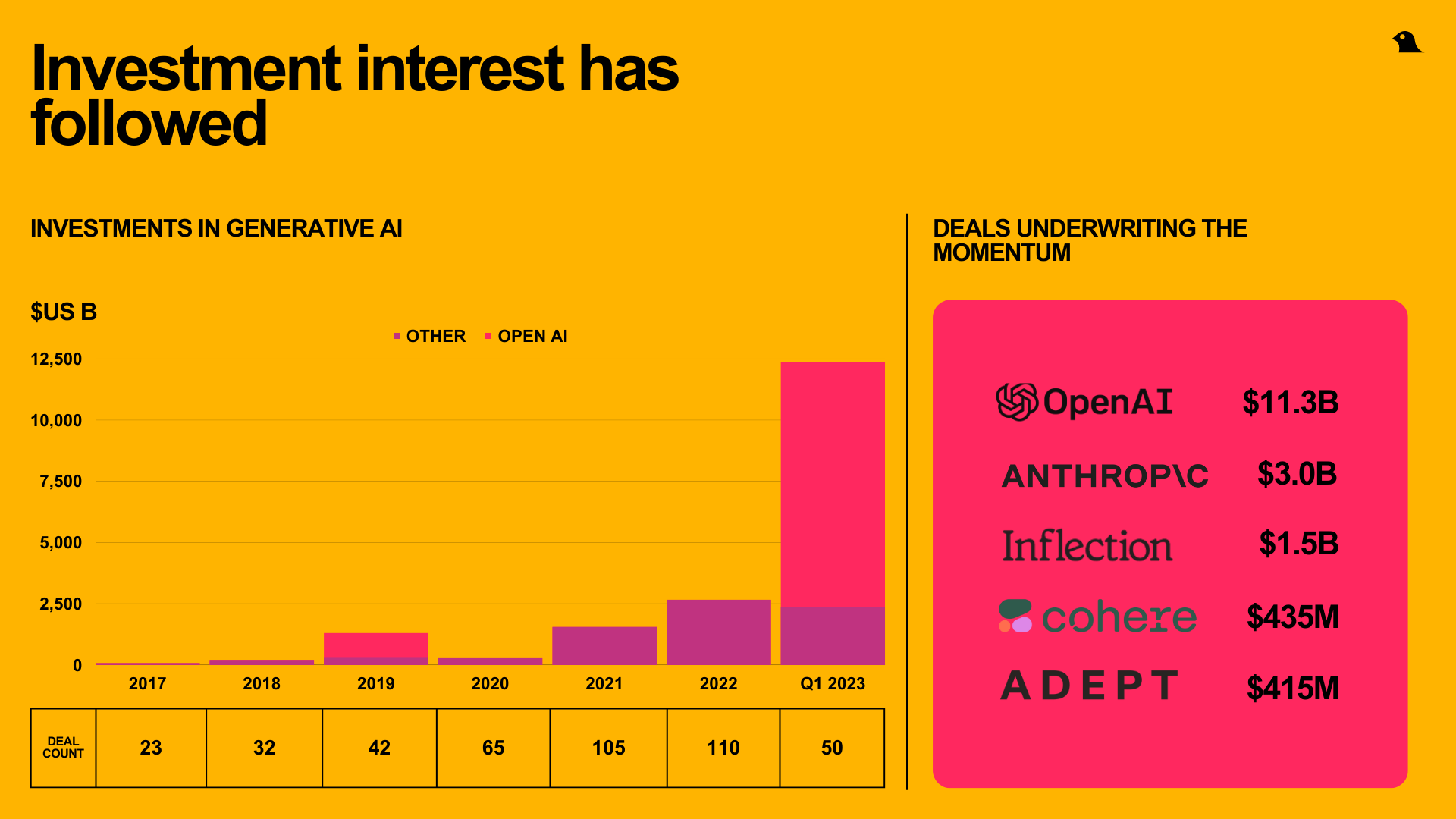
Disruption is varied
For the first time, technology advancements are disproportionately affecting white-collar jobs, like engineers, marketers and lawyers; whereas the Industrial Revolution and Information Age impacted blue-collar productivity, marked by distinct periods of digitisation and manufacturing automation.
The AI value proposition for knowledge workers is compelling, increasing productivity and effectiveness (easily outperforming humans on many tasks now), and lowering costs.
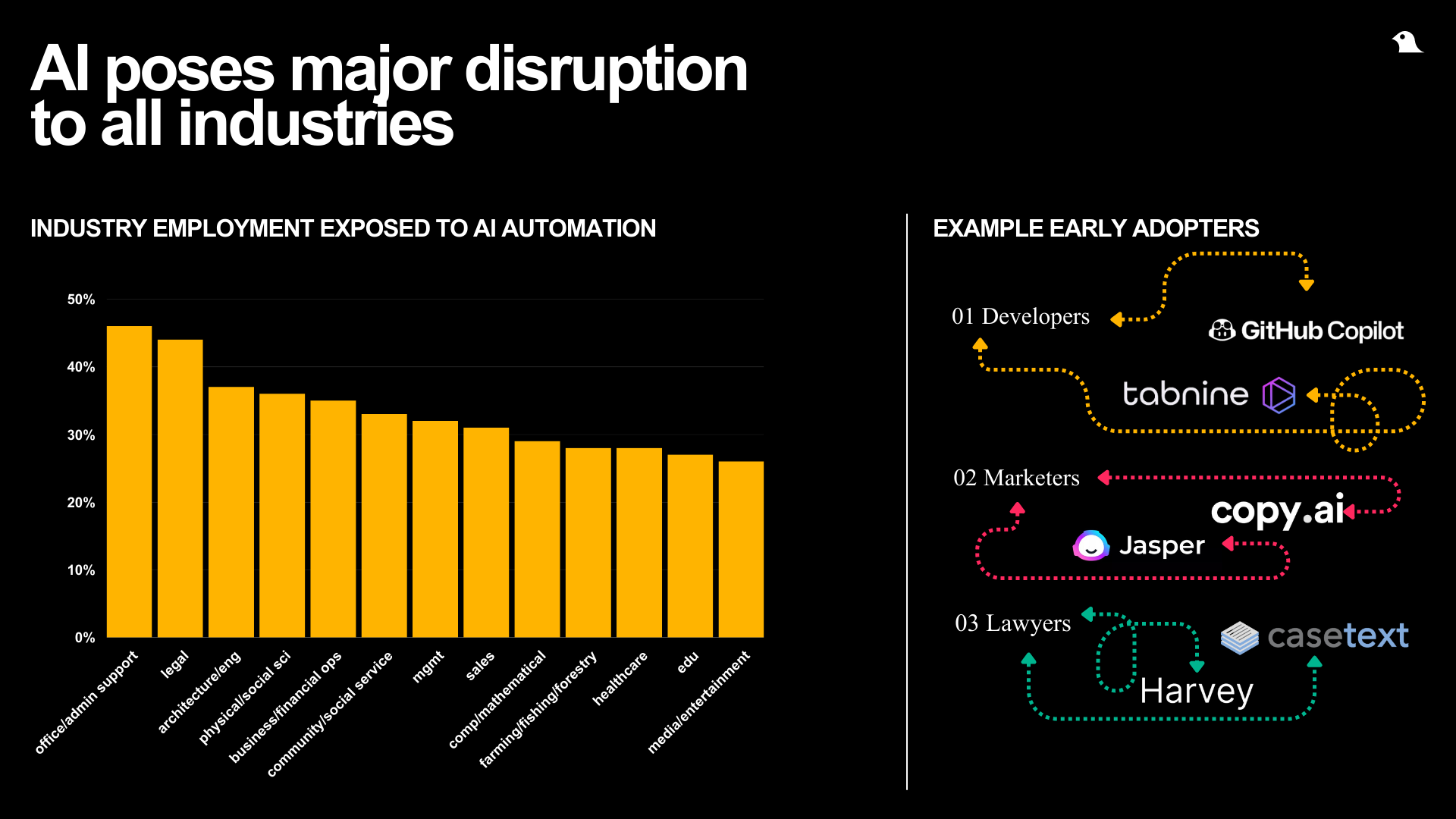
All industries are experiencing, or will experience, disruption to varying degrees and on different timelines.
Some are early adopters:
Customer Support - LLMs are well suited to the conversational, chat-based nature of support and incumbents are making moves. Intercom launched Fin AI Resolutions which automates resolution for 50% of support queries with a sticker price of $0.99 per ticket.
Marketing - Jasper, Typeface, Copy.ai were quick out of the gate to attack the marketing function, but now face defensibility questions as their initial advantage was reliant on quickly spinning out applications from foundational models.
Engineering - Github launched Copilot in June 2021, built on top of Codex which trained GPT on 54 million Github code repositories. Others such as Codium and Tabnine have emerged in the category too.
In December 2022, we hosted the CEO of Github Thomas Dohmke in Sydney for a fireside chat, where he shared his thoughts on Copilot:
“A lot of what developers are writing is predictable and not that creative. It is the easiest pitch ever to customers, we can see 40% of code is AI-written in the files where Copilot is enabled.
If you tell a VP of Engineering or CTO that 40% of their code can be written by AI and what that does for your engineering productivity, they are all like "wow, we've been talking about making developers more productive for years” – that was the whole idea behind Agile, Kanban, DevOps in general – but that promise has not truly been delivered; it hasn’t helped with developer satisfaction or made them happier. AI does.
We’re here in Australia, let’s take the analogy is surfing, AI helps developers focus all their time on flow and riding waves, and takes on all the drudgery like, falling in the water and paddling back out!"
Other industries will take longer to adopt. Healthcare and law are moving fast but are areas where regulation is high (e.g. HIPAA), syntax is specific, accuracy is critical (given the risk of hallucinations), and there is significant opportunity for improving foundational models.
It has been an historic year for AI, from the pace of progress to the amount of capital being poured into applications and infrastructure. One thing is for certain, AI is profoundly changing the way we work.
______
Coming next
Part 2: Landscape - a review of the AI landscape and where the emerging pockets of opportunity for investment are.
Part 3: Investment Thesis - an overview of our mental model for assessing AI companies.
Part 4: Company Spotlights - case studies from within our portfolio and noteworthy external examples.
In the meantime, we'd love to hear your thoughts. You can find Silk on LinkedIn and X, and Tom on LinkedIn and X.
P.S.
If you were wondering, this blog was not written using generative AI... or was it? Case in point.
Our banner image on the other hand was generated using Leonardo.ai, showing us what the Blackbird Team would look like as Pixar characters!
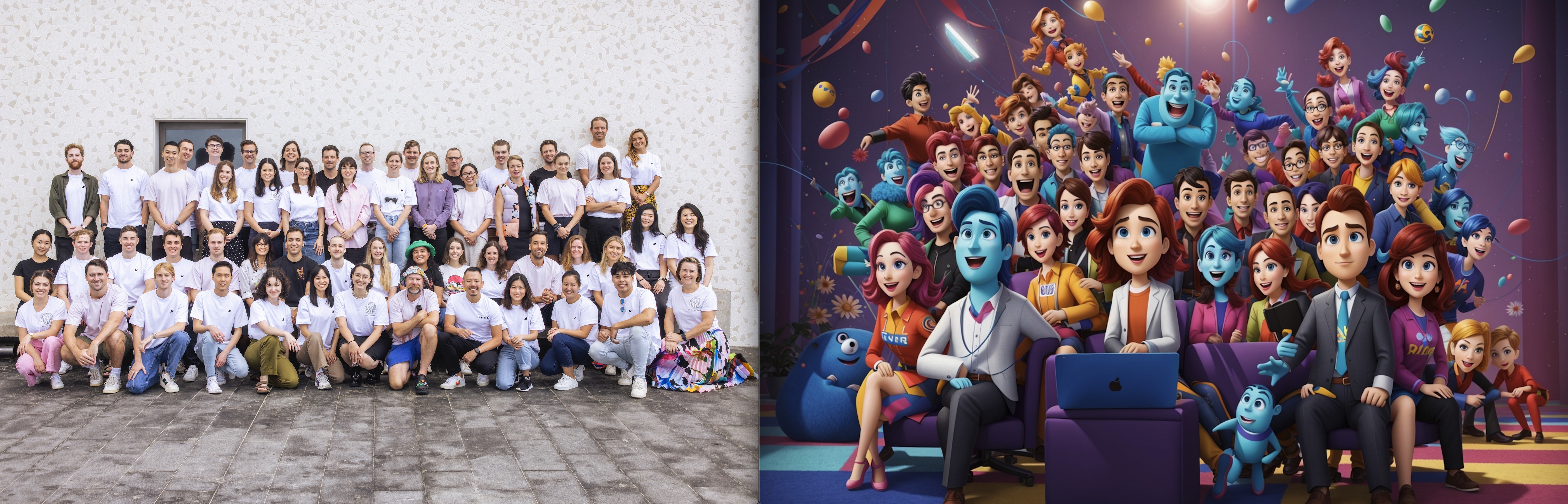