.avif)
Indistinguishable from Magic: The 7 Sources of Moat in AI
“In business, I look for economic castles protected by unbreachable moats” - Warren Buffet
Welcome to Part 3 of a 4-part series on the state of AI and our emerging perspectives.
In Part 1 we took stock of the past year and the speed and scale of the developing AI market. In Part 2 we walked through the AI market map and highlighted pockets of opportunity. Now, in Part 3 we share insights into what we look for in AI companies and our mental model for how we think about AI companies building sustainable competitive advantage, or moat.
Part 3: The 7 Sources of Moat in AI
Cautionary tales of “GPT Wrappers”
There are oodles of blogs, articles and thought pieces on the need for “defensibility”, “sustainable advantage” or “competitive moat” in AI (example, example, example, example).
And there are cautionary tales of “GPT Wrappers”, products with a thin surface layer built on top of third party model APIs, which lack enough unique product depth and IP to protect against fast-copies.
An often referenced case study is Jasper AI. Jasper took off early and aggressively, shooting from basically nothing to U$42M ARR in its first year and ~U$72M in its second. Around that time, in October 2022, it raised an impressive U$125M Series A round at a $1.5B valuation.
.avif)
Jasper’s initial growth was underscored by being a first mover and the fact it got early access to GPT-3 at a time when the API was closed to the broader market.
But then it stumbled.
In November 2022, one month following the Series A raise announcement, OpenAI released GPT-3.5 along with its free ChatGPT user application. ChatGPT grew rapidly gaining 100M MAUs in 2 months, the fastest acquisition of users of any tech product in history, and it could fulfil many of the use cases that Jasper served, for free.
In the same month, OpenAI switched its API to be openly accessible which resulted in many fast-followers to the generative copy opportunity (Copy.ai, Grammarly, Quillbot, Copysmith, Notion AI, Clickup AI, Hypotenuse, Writesonice, Wordtune, Rytr).
Jasper had a great product but a fairly thin layer of moat. It was using an off-the-shelf LLM that was delivering a significant proportion of product value for its primary use cases, marketing copy, without much room for fine-tuning, the rest of the product e.g. user workflows and editing features, had not yet been fully fleshed out.
To its credit, Jasper is well capitalised, has built a large community, seems to be investing heavily in product and LLM capabilities, and still has a shot at success… but the point remains, moat is important.
7 Sources of Moat
The risk of the “GPT Wrapper” naturally gives rise to the question of what is moat and how do you build it?
Many say “it’s about owning the data”. In reality, there are multiple levers for building moat and the one you should pull on depends on your product strategy and use case.
In our experience to date, there are seven core sources of moat in AI, let’s explore each of these.
.avif)
Moat 1 - The Product Layer
Recent advancements in AI have led to a resurgence in vertical and horizontal SaaS solutions. Historically, these solutions have focused on workflows, with powerful AI just an API away. They now can play a big role in automation too, increasing their value propositions, ACVs (Average Contract Values) and TAMs (Total Addressable Markets).
AI has a different role to play depending on the product and vertical. Customer support products (e.g. Intercom, Zendesk) are being easily disrupted given the opportunity for AI to enable the core user need i.e. effective, chat-based resolution of user support tickets. Products in other verticals like manufacturing and construction may find this disruption is less pronounced.
A helpful question to ask is “how much of the product’s value proposition is or can be derived from AI-based features?”. The answer might be 50%+ (the product is AI-dominant), 25-50% (the product is AI-core), 10-25% (the product is AI-enabled) or ~5-10% (the product is AI-assisted).
.avif)
The greater the role of AI in the product (i.e. AI-dominant or -core), the more important it is to understand the infrastructure stack and where the long-term moat may come from. Alternatively, if there is significant value that comes from the broader non-AI features (e.g. AI-assisted and -enabled), the greater the opportunity for moat to exist at the product layer.
Take Dovetail, the enterprise platform for customer insights. Opportunities for applying AI within the product are extensive, for example, to auto-tag videos, generate summaries of interview transcripts, or automate themes and analysis. See a summary of Dovetail’s AI features here, yet beyond these features, Dovetail has a deep wealth of product value i.e. an entire workflow solution for user research teams to capture and store insights, collaborate, analyse, report on data, and scale research. It is an AI-core company and is using AI infrastructure while maintaining its moat at the product layer.
This is not to say that incumbents with broader products in the AI-assisted or AI-enabled categories will always win. AI may only be a part of the product but be so disruptive that the entire UI needs to be rearchitected from the ground up, turning incumbent product frameworks into tech debt.
Moat 2 - Proprietary Data
“Data is the new oil” and with advances in AI, data is now the best form of crude. Data serves as the lifeblood for training models from scratch or the fine-tuning of foundational models.
Proprietary data is far and away the most talked about source of moat in venture. Even notoriously open companies have begun to close access to their data e.g. Reddit and X have shut down their APIs. In Part 2, we discussed how we expect to see more data-as-a-service models emerge where companies sell access to their data as a model input.
Many companies have built moat off the back of access to proprietary data for model development. For example, Github Copilot (code automation for developers using an AI model called Codex trained on 54M Github code repositories), BloombergGPT (a specialised LLM trained on Bloomberg’s proprietary financial data), or Grok (built by X.ai and trained off the data of X fka Twitter).
In the Blackbird portfolio, Harrison AI partnered with i-Med to gain access to one of the world’s largest medical imaging datasets and build proprietary models behind products like Annalise AI. Annalise is a radiologist’s copilot, trained on 10B images, it runs software that can detect hundreds of different clinical findings (or cancerous anomalies) in chest X-rays and brain CT scans.
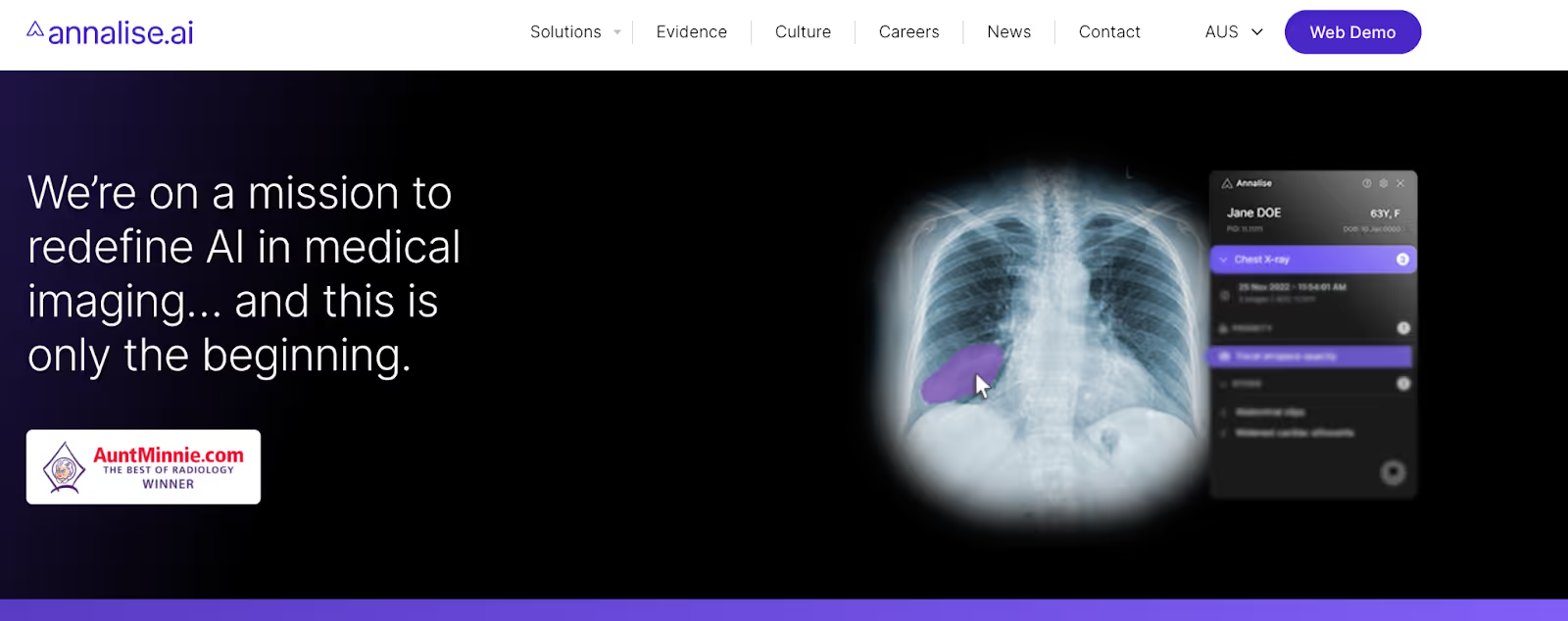
The role of proprietary data in AI can give rise to what may seem to be niche use cases. Evenup for example is focused purely on personal injury law, it has trained its models on “250K+ public verdicts and private settlements” and recently raised a U$51M Series B.
AI is not just breathing life back into vertical SaaS as a category but is giving rise to new “hyper-vertical” opportunities.
The value of proprietary data as a moat is a function of:
1) The scarcity of access to the type of data.
2) The scale of the specific AI use case.
3) The weakness in performance of more generalised AI models for that use case.
4) The importance of model accuracy, healthcare being a prime example.
Moat 3 - Data Interpretation
Having access to data is one thing, understanding it is another.
Some data types are complex in structure and context. Take presentations, context does not just come from the words on the slides but also the shapes, headings, images and layout. If you were to just take all the words from the presentation and pump them through an AI model, the context would be lost.
Some industries have unique syntax and terminology creating the opportunity to fine-tune and train models to understand those nuances.
Complexities give rise to a breed of moat where the secret sauce is not necessarily in having proprietary access to data but in building the unique capabilities for processing and interpreting it.
Good examples are companies building in the receipt processing category such as Brex. Receipts can vary widely in format, Brex has done a substantial amount of human labelling and feeds in data from calendar entries, organisational context, previous expenses, and exclusive integrations to enhance context and improve the accuracy of data interpretation.
.avif)
Or Runway, which is focused on building AI models for video and motion pictures. Wrangling video as a medium is hard, Runway’s interpreting capabilities of the complexity of the underlying data, is their moat.
Moat 4 - Prompt Engineering
Putting aside AI models and the data used to train or fine-tune them, valuable insights can also be derived to guide the model prompt process.
A prompt is the instructions sent to an AI model to generate an output, and prompt engineering is the practice of adjusting or tailoring instructions for the optimal response. This process gives rise to another source of moat.
Let’s use Canva and Midjourney to illustrate the point. Say you are putting together a presentation for work using Canva on the topic of a new HR policy and want to add an image to one of your slides of a “kitten lying in the sun purring”. First, you go to Midjourney and process that prompt and it returns four images. Then you go to Canva and process the same prompt, here however, Canva knows a lot more about what is going on. It knows:
1) The exact context of the presentation you are working on (the HR policy update).
2) The style preferences of the presentation (colour scheme, image and design parameters).
3) The user preferences from past presentations (perhaps even feedback received on those past presentations).
This additional data is incredibly valuable and can provide context to engineer the prompt sent to the model and increase the likelihood that the images returned hit the mark.
.avif)
Glean has built an enterprise AI search and knowledge discovery platform that sits on top of data sourced through integrations with major workplace systems like Google Drive, Slack, Zoom, Outlook and Jira. Here, the sum is greater than the parts, by sitting across all systems Glean can engineer user prompts 10x more effectively than an AI search feature built on top of the data of each system alone. Having more data leads to better prompts.
Moat 5 - Model Evaluation
Model evaluation is one of the more exciting areas of moat despite being less talked about.
Model evaluation is the process of using evaluation techniques and metrics to understand the performance of a machine learning model, its strengths and weaknesses.
There are different methods of evaluation, for example:
- Human feedback: when a model provides a user with a response, the user can rate the response.
- Manual review: have staff or professionals review and rate the quality of responses.
- Standardised tests: often generalised models are fed tests like the GAMSAT to test the number of questions answered correctly.
- Product analytics: A/B testing different responses or gauging performance from the actions taken by the user following the response.
Effective model evaluation is emerging as a key source of moat, ranking model output is a reinforcing input back into the model. Right now, closed-source model providers tend to have better evaluation processes than open-source providers, and use these evaluations to create reward schemas for their models.
Using legal AI as an example, say you receive a contract from a supplier and want to review the clauses, you upload the contract to a standalone legal AI application and it gives you suggestions for how to adjust the NDA clause to be a mutual NDA. You swap out the terms, send the contract back to the supplier and the negotiation continues.
Alternatively, you use Ironclad which builds software for contract management. You upload the contract and it suggests how you could adjust the NDA clause to be a mutual NDA but those suggestions are enhanced by past performance insights e.g. “if you use this version of the term, we estimate a 95% chance that the other party will accept this within 3 days”.
Because Ironclad manages the full contracting cycle between parties, over time, it builds a unique feedback loop of insight into which terms get pushed back on or accepted, who accepts terms or rejects, how long acceptance takes etc and this is powerful.
Moat 6 - Product UX
The product UX that wraps around an AI application or feature is crucial to get right and a source of moat.
Many applications are developing as human-in-the-loop copilots but how you “loop” in the human is important. Github pioneered the copilot model with Github Copilot which seamlessly integrates into the developer workflow in a non-intrusive way. Compared to ChatGPT, which has a pretty clunky UX i.e. opens on a separate webpage, type into a text box, get a response, and copy/paste it to where it needs to go.
Incumbent solutions can carry tech debt which makes it hard to simply integrate or tack-on AI features, the optimal product UX for an AI application might need to be completely re-architected.
This concept was articulated in a recent Sacra article using the example of Microsoft Clippy:
“While Microsoft Office, Google Workspace and Adobe Creative Cloud have the power of distribution and bundlenomics, non-AI native incumbents risk stapling AI on as a "feature" à la Microsoft Clippy rather than weaving AI into the foundation of the products.
Incumbents are constrained by their existing product design and are at a disadvantage compared to AI-native apps when it comes to building a cohesive, end-to-end AI product experience.”
Leonardo.ai and Midjourney are image generation platforms. Midjourney’s UX is based in Discord, users post, somewhat awkwardly, prompts in public channels on Discord and the platform has almost no prompt or editing controls. Leonardo has built a beautiful web-based experience with powerful editing features, custom AI models, style preferences, and workflows, giving the user deeper interaction with the AI experience.
.png)
Moat 7 - Orchestration and Tooling
The final source of moat is in the orchestration and tooling stack. Here we are talking about companies that are building unique advantages through the ways in which they approach AI infrastructure and optimise for cost and performance (e.g. speed/latency).
One of the biggest challenges is that margins tend to be slim and the infrastructure complex and hard to scale. Running training and inference is expensive, mounting spend required for GPUs, compute and third party services (such as GPT-4 API calls from OpenAI). Scalability is also a challenge, OpenAI had to turn off Pro subscriptions due to excess demand and an inability to scale their infrastructure fast enough.
Avatar AI and Lensa AI are another interesting case study. Avatar and Lensa launched about a month apart in October and November 2022 with apps that allowed users to create AI avatars from a selfie.
Avatar peaked at U$22K in daily revenue and quickly dropped off. Lensa took off and hit over $30M revenue by December 2022. Core to this difference, was the way in which Lensa approached its infrastructure, Lensa built to be scalable and 10x cheaper which allowed them to charge the end user a lower fee (~40% less than Avatar), make more margin and keep up with the rapid onslaught of demand.
.avif)
While both apps did not have a long-term moat (i.e. they were both a thin wrapper built on Stable Diffusion), or sustainable demand, their underlying choices in architecture defined success.
Some companies manage cost through prompt triaging and optimising how they direct prompts through different models, maximising for prompts sent through open-source models and only escalating to more expensive models like GPT-4 where the type of prompt or performance requires it.
On the training side, Strong Compute is building its business off the back of a value proposition to support companies in managing their model training costs.
Other companies are taking it a step further and managing cost through model distillation, training free open-source models on the output from closed-sourced models to elevate the overall performance of the OS model. This is a little cheeky and could breach provider licences.
However, outside of cost, many companies are building infrastructure moats through better performance. Marqo’s secret sauce lies in their technical scalability and speed of processing real-time data for use cases that value latency.
Summary
The progress achieved over the past year is astounding but we have a long way to go before the widespread adoption and wielding of AI’s capabilities, which creates a wealth of blue ocean opportunity.
While there are other levers that companies can pull on to build moat beyond those that are specific to AI, like network effects, scale effects, regulatory, integrations, and brand, for now, we see these 7 sources of moat (that can be built in isolation or combination) as the prevailing areas for companies building in AI to develop and enhance sustainable advantage over time.
Coming up next is Part 4, where we highlight AI case studies from within our portfolio and noteworthy external examples.
A special thanks to Alex Edwards for his help on this piece.